Managing Python environments and Jupyter kernels: Difference between revisions
No edit summary |
|||
(77 intermediate revisions by 6 users not shown) | |||
Line 1: | Line 1: | ||
{{obsolete|software/conda_environments/}} | |||
[[Category:Python]] | |||
{|align=right | |||
|__TOC__ | |||
|} | |||
{{:Conda}} | |||
== Install packages into your environment with mamba or pip == | |||
<div class="mw-collapsible mw-collapsed" style="width:70%; padding: 5px; border: 1px solid gray;"> | <div class="mw-collapsible mw-collapsed" style="width:70%; padding: 5px; border: 1px solid gray;"> | ||
''Expand this section to view instructions | ''Expand this section to view instructions.'' | ||
<div class="mw-collapsible-content" style="padding: 5px;"> | <div class="mw-collapsible-content" style="padding: 5px;"> | ||
Now we are ready to start adding things to our environment. | Now we are ready to start adding things to our environment. | ||
Line 144: | Line 16: | ||
{{Note| '''Note:''' when an environment is active, running <code>pip install</code> will install the package ''into that environment''. So, even if you continue using <code>pip</code>, adding <code>conda</code> environments solves the problem of everything being installed in one location--each environment has its own <code>site-packages</code> folder and is isolated from other environments.|note}} | {{Note| '''Note:''' when an environment is active, running <code>pip install</code> will install the package ''into that environment''. So, even if you continue using <code>pip</code>, adding <code>conda</code> environments solves the problem of everything being installed in one location--each environment has its own <code>site-packages</code> folder and is isolated from other environments.|note}} | ||
'''<big><code>mamba install</code> packages</big>''' | |||
Now we are ready to install packages using <code>mamba install ___</code>. | Now we are ready to install packages using <code>mamba install ___</code>. | ||
'''Start with <code>cudatoolkit</code> and </code>pytorch</code>/<code>tensorflow</code> if using GPU!''' | |||
{{Note| If you | {{Note|'''If you plan on using a GPU''' see below|warn}} | ||
To make sure your code will run on GPUs install a recent <code>cudatoolkit</code> package that works with the NVIDIA drivers on HPG (currently 12.x, but older versions are still supported) alongside the pytorch or tensorflow package(s). See RC provided tensorflow or pytorch installs for examples if needed. Mamba can detect if there is a gpu in the environment, so the easiest approach is to run the mamba install command in a [https://help.rc.ufl.edu/doc/GPU_Access gpu session]. Alternatively, you can run mamba install on any node or if a cpu-only pytorch package was already installed by explicitly requiring a gpu version of pytorch when running mamba install. E.g. | |||
mamba install cudatoolkit=11.3 pytorch pytorch-cuda=11.3 -c pytorch -c nvidia | |||
'''<big>Load the <code>conda</code> module</big>''' | |||
From the [https://pytorch.org/get-started/locally/ PyTorch Installation page], we should use: | From the [https://pytorch.org/get-started/locally/ PyTorch Installation page], we should use: | ||
Line 158: | Line 31: | ||
<code>mamba install pytorch torchvision torchaudio cudatoolkit=11.3 -c pytorch</code> | <code>mamba install pytorch torchvision torchaudio cudatoolkit=11.3 -c pytorch</code> | ||
When you run that command, <code>mamba</code> will look in the repositories for the specified packages and their dependencies. Note we are specifying a particular version of <code>cudatoolkit</code>. As of May, 2022, that is the correct version on HiPerGator. Here's a screenshot of part of the output: | When you run that command, <code>mamba</code> will look in the repositories for the specified packages and their dependencies. Note we are specifying a particular version of <code>cudatoolkit</code>. As of May, 2022, that is the correct version on HiPerGator. | ||
[[File:Mamba install.png|frameless | <div class="mw-collapsible mw-collapsed" style="width:70%; padding: 5px; border: 1px solid gray;"> | ||
''Here's a screenshot of part of the output:'' | |||
<div class="mw-collapsible-content" style="padding: 5px;"> | |||
[[File:Mamba install.png|frameless]] | |||
</div> | |||
</div> | |||
<code>mamba</code> will list the packages it will install and ask you to confirm the changes. Typing 'y' or hitting return will proceed; 'n' will cancel: | <code>mamba</code> will list the packages it will install and ask you to confirm the changes. Typing 'y' or hitting return will proceed; 'n' will cancel: | ||
Finally, <code>mamba</code> will summarize the results: | Finally, <code>mamba</code> will summarize the results: | ||
Line 169: | Line 45: | ||
[[File:Mamba success.png|frameless|center|600px]] | [[File:Mamba success.png|frameless|center|600px]] | ||
'''Tensorflow installation alternative''' | |||
While not needed for this tutorial, many users will want TensorFlow instead of PyTorch, so we will provide the command for that here. To install TensorFlow, use this command: | While not needed for this tutorial, many users will want TensorFlow instead of PyTorch, so we will provide the command for that here. To install TensorFlow, use this command: | ||
<code>mamba install tensorflow cudatoolkit | <code>mamba install tensorflow cudatoolkit=11.2</code> | ||
This post at conda-forge has additional information and tips for installing particular versions or installing on a non-GPU node: [https://conda-forge.org/blog/posts/2021-11-03-tensorflow-gpu/ GPU enabled TensorFlow builds on conda-forge]. | This post at conda-forge has additional information and tips for installing particular versions or installing on a non-GPU node: [https://conda-forge.org/blog/posts/2021-11-03-tensorflow-gpu/ GPU enabled TensorFlow builds on conda-forge]. | ||
'''<big>Install additional packages</big>''' | |||
This tutorial creates an environment for the [https://github.com/huggingface/deep-rl-class Hugging Face Deep Reinforcement Learning Course], you can either follow along with that or adapt to your needs. | This tutorial creates an environment for the [https://github.com/huggingface/deep-rl-class Hugging Face Deep Reinforcement Learning Course], you can either follow along with that or adapt to your needs. | ||
Line 185: | Line 61: | ||
<code>mamba install gym-box2d stable-baselines3</code> | <code>mamba install gym-box2d stable-baselines3</code> | ||
'''<big>Add packages to our environment with <code>pip install</code></big>''' | |||
As noted above, not everything is available in a <code>conda</code> channel. For example the next thing we want to install is <code>huggingface_sb3</code>. | As noted above, not everything is available in a <code>conda</code> channel. For example the next thing we want to install is <code>huggingface_sb3</code>. | ||
If we type <code>mamba install huggingface_sb3</code>, we get a message saying nothing provides it: | If we type <code>mamba install huggingface_sb3</code>, we get a message saying nothing provides it as seen to the right: | ||
[[File:Mamba not available.png|382px|frameless| | [[File:Mamba not available.png|382px|frameless|right]] | ||
If we know of a <code> | If we know of a <code>conda</code> source that has that package, we can add it to the <code>channels:</code> section of our <code>~/.condarc</code> file. That will prompt <code>mamba</code> to include that location when searching. | ||
But many things are only available via <code>pip</code>. So... | But many things are only available via <code>pip</code>. So... | ||
Line 201: | Line 77: | ||
That will install <code>huggingface_sb3</code>. Again, because we are using environments and have the <code>hfrl</code> environment active, <code>pip</code> will not install <code>huggingface_sb3</code> in our </code>~/.local/lib/python3.X/site-packages/</code> directory, but rather within in our <code>hfrl</code> directory, at <code>/blue/group/user/conda/envs/hfrl/lib/python3.10/site-packages</code>. This prevents the issues and headaches mentioned at the start. | That will install <code>huggingface_sb3</code>. Again, because we are using environments and have the <code>hfrl</code> environment active, <code>pip</code> will not install <code>huggingface_sb3</code> in our </code>~/.local/lib/python3.X/site-packages/</code> directory, but rather within in our <code>hfrl</code> directory, at <code>/blue/group/user/conda/envs/hfrl/lib/python3.10/site-packages</code>. This prevents the issues and headaches mentioned at the start. | ||
'''<big>Install additional packages</big>''' | |||
As with <code>mamba</code>, we could list multiple packages in the <code>pip install</code> command, but again, we only need one more: | As with <code>mamba</code>, we could list multiple packages in the <code>pip install</code> command, but again, we only need one more: | ||
<code>pip install ale-py==0.7.4</code> | <code>pip install ale-py==0.7.4</code> | ||
</div></div> | |||
= Use your | == Use your environment from command line or scripts == | ||
Now that we have our environment ready, we can use it from the command line or a script using something like: | Now that we have our environment ready, we can use it from the command line or a script using something like: | ||
{|cellpadding="10" | |||
|-style="vertical-align:top;" | |||
| | |||
<pre> | <pre> | ||
module load conda | module load conda | ||
Line 218: | Line 97: | ||
python amazing_script.py | python amazing_script.py | ||
</pre> | </pre> | ||
|| | |||
or with ''path'' based environments: | or with ''path'' based environments: | ||
|| | |||
<pre> | <pre> | ||
# Set path to environment we want and pre-pend to PATH variable | |||
env_path=/blue/ | env_path=/blue/mygroup/share/project42/conda/bin | ||
export PATH = | export PATH=$env_path:$PATH | ||
# Run my amazing python script | # Run my amazing python script | ||
python amazing_script.py | python amazing_script.py | ||
</pre> | </pre> | ||
|} | |||
== Setup a Jupyter Kernel for your environment == | |||
<div class="mw-collapsible mw-collapsed" style="width:70%; padding: 5px; border: 1px solid gray;"> | |||
''Expand this section to view instructions.'' | |||
<div class="mw-collapsible-content" style="padding: 5px;"> | |||
Often, we want to use the environment in a [[Jupyter_Notebooks|Jupyter notebook]]. To do that, we can create our own Jupyter Kernel. | |||
'''<big>Add the <code>jupyterlab</code> package</big>''' | |||
In order to use an environment in Jupyter, we need to make sure we install the <code>jupyterlab</code> package in the environment: | |||
<code>mamba install jupyterlab</code> | |||
'''<big>Copy the <code>template_kernel</code> folder to your path</big>''' | |||
On HiPerGator, Jupyter looks in two places for kernels when you launch a notebook: | |||
# <code>/apps/jupyterhub/kernels/</code> for the globally available kernels that all users can use. (Also a good place to look for troubleshooting getting your own kernel going) | |||
# <code>~/.local/share/jupyter/kernels</code> for each user. (Again, your home directory and the <code>.local</code> folder is hidden since it starts with a dot) | |||
Make the <code>~/.local/share/jupyter/kernels</code> directory: <code>mkdir -p ~/.local/share/jupyter/kernels</code> | |||
Copy the <code>/apps/jupyterhub/template_kernel</code> folder into your <code>~/.local/share/jupyter/kernels</code> directory: | |||
<code>cp -r /apps/jupyterhub/template_kernel/ ~/.local/share/jupyter/kernels/hfrl</code> | |||
{{Note|'''Note:''' This also renames the folder in the copy. It is important that the directory names be distinct in both your directory and the global <code>/apps/jupyterhub/kernels/</code> directory.|note}} | |||
'''<big>Edit the <code>template_kernel</code> files</big>''' | |||
The <code>template_kernel</code> directory has four files: the <code>run.sh</code> and <code>kernel.json</code> files will need to be edited in a text editor. We will use <code>nano</code> in this tutorial. The <code>logo-64X64.png</code> and <code>logo-32X32.png</code> are icons for your kernel to help visually distinguish it from others. You can upload icons of those dimensions to replace the files, but they need to be named with those names. | |||
'''Edit the <code>kernel.json</code> file''' | |||
Let's start editing the <code>kernel.json</code> file. As an example, we can use: | |||
<code>nano ~/.local/share/jupyter/kernels/hfrl/kernel.json</code> | |||
The template has most of the information and notes on what needs to be updated. Edit the file to look like: | |||
<pre> | |||
{ | |||
"language": "python", | |||
"display_name": "HF_Deep_RL", | |||
"argv": [ | |||
"~/.local/share/jupyter/kernels/hfrl/run.sh", | |||
"-f", | |||
"{connection_file}" | |||
] | |||
} | |||
</pre> | |||
'''Edit the <code>run.sh</code> file''' | |||
The <code>run.sh</code> file needs the path to the <code>python</code> application that is in our environment. The easiest way to get that is to make sure the environment is activated and run the command: <code>which python</code> | |||
The path it outputs should look something like: <code>/blue/group/user/conda/envs/hfrl/bin/python</code>. Copy that path. | |||
Edit the <code>run.sh</code> file with <code>nano</code>: | |||
<code>nano ~/.local/share/jupyter/kernels/hfrl/run.sh</code> | |||
The file should looks like this, '''but with your path''': | |||
<pre> | |||
#!/usr/bin/bash | |||
exec /blue/ufhpc/magitz/conda/envs/hfrl/bin/python -m ipykernel "$@" | |||
</pre> | |||
If you are doing this in a Jupyter session, refresh your page. If not, launch Jupyter. | |||
Your kernel should be available in the default kernel list ready for you to use! | |||
</div></div> |
Latest revision as of 01:57, 10 January 2025
This page is obsolete. It is being retained for archival purposes. The current version of this page can be found at https://docs.rc.ufl.edu/software/conda_environments/
Many projects that use Python code require careful management of the respective Python environments. Rapid changes in package dependencies, package version conflicts, deprecation of APIs (function calls) by individual projects, and obsolescence of system drivers and libraries make it virtually impossible to use an arbitrary set of packages or create one all-encompassing environment that will serve everyone's needs over long periods of time. The high velocity of changes in the popular ML/DL frameworks and packages and GPU computing exacerbates the problem.
The problem with pip install
Expand this section to view pip problems and how conda/mamba mends them.
Most guides and project documentation for installing python packages recommend using pip install
for package installation. While pip
is easy to use and works for many use cases, there are some major drawbacks. There are a few issues with doing pip install
on a supercomputer like HiPerGator:
- Pip by default installs binary packages (wheels), which are often built on systems incompatible with HiPerGator. This can lead to importing errors, and its attempts to build from source will fail without additional configuration.
- If you are pip installing a package that is/will be installed in an environment provided by UFRC, your pip version will take precedence. Your dependencies eventually become incompatible causing errors, with even one pip install making environments unusable.
- Different packages may require different versions of the same package as dependencies leading to impossible to reconcile installation scenarios. This becomes a challenge to manage with
pip
as there isn't a method to swap active versions. - On its own, `pip` installs **everything** in one location:
~/.local/lib/python3.X/site-packages/
.
Conda and Mamba to the rescue!
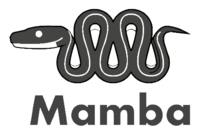
conda
and the newer, faster, drop-in replacement mamba
, were written to solve some of these issues. They represent a higher level of packaging abstraction that can combine compiled packages, applications, and libraries as well as pip-installed python packages. They also allow easier management of project-specific environments and switching between environments as needed. They make it much easier to report the exact configuration of packages in an environment, facilitating reproducibility. Moreover, conda environments don't even have to be activated to be used; in most cases adding the path to the conda environment's 'bin' directory to the $PATH in the shell environment is sufficient for using them.
A caveat
conda
and mamba
get packages from channels, or repositories of prebuilt packages. While there are several available channels, like the conda-forge
or bioconda
, not every Python package is available from such channel as they have to be packaged for conda first. You may still need to use pip
to install some packages as noted later. However, conda
still helps manage environment by installing packages into separate directory trees rather than trying to install all packages into a single folder that pip does.
Configuration
Expand this section to view instructions for configuring Conda
The ~/.condarc
configuration file
conda
's behavior is controlled by a configuration file in your home directory called .condarc
. The dot at the start of the name means that the file is hidden from 'ls' file listing command by default. If you have not run conda
before, you won't have this file. Whether the file exists or not, the steps here will help you modify the file to work best on HiPerGator. First load of the conda
environment module on HiPerGator will put the current best practice .condarc
into your home directory.
conda
package cache location
conda
caches (keeps a copy) of all downloaded packages by default in the ~/.conda/pkgs
directory tree. If you install a lot of packages you may end up filling up your home quota. You can change the default package cache path. To do so, add or change the pkgs_dirs
setting in your ~/.condarc
configuration file e.g.:
pkgs_dirs: - /blue/mygroup/$USER/conda/pkgs
Replace mygroup
with your actual group name.
conda
environment location
conda
puts all packages installed in a particular environment into a single directory. By default named conda
environments are created in the ~/.conda/envs
directory tree. They can quickly grow in size and, especially if you have many environments, fill the 40GB home directory quota. For example, the environment we will create in this training is 5.3GB in size. As such, it is important to use path based (conda create -p PATH) conda environments, which allow you to use any path for a particular environment for example allowing you to keep a project-specific conda environment close to the project data in /blue/ where you group has terrabyte(s) of space.
You can also change the default path for the name environments (conda create -n NAME
) if you prefer to keep all conda
environments in the same directory tree. To do so, add or change the envs_dirs
setting in the ~/.condarc
configuration file e.g.:
envs_dirs: - /blue/mygroup/share/conda/envs #or alternatively: - /blue/mygroup/$USER/conda/envs
Replace mygroup
with your actual group name.
One way to edit your ~/.condarc
file is to type: nano ~/.condarc`
If the file is empty, paste in the text below, editing the env_dirs:
and pkg_dirs
as below. If the file has contents, update those lines.
~/.condarc
should look something like this when you are done editing (again, replacing group
and user
in the paths with your group and username).channels: - conda-forge - bioconda - defaults envs_dirs: - /blue/group/user/conda/envs pkgs_dirs: - /blue/group/user/conda/pkgs auto_activate_base: false auto_update_conda: false always_yes: false show_channel_urls: false
Create and activate a Conda environment
Expand this section to view instructions for setting up environments.
UF Research Computing Applications Team uses conda for many application installs behind the scenes. We are happy to install applications on request for you. However, if you would like to use conda to create multiple environments for your personal projects we encourage you to do so. Here are some recommendations for successful conda use on HiPerGator.
- See https://docs.conda.io/projects/conda/en/latest/user-guide/tasks/manage-environments.html for the original documentation on managing conda environments.
- We recommend creating environments by 'path', so they won't fill up your home directory (check quota with home_quota). The resulting environment should be located in the project(s) directory tree in /blue for better tracking of installs and better filesystem performance compared to home.
To make sure your code will run on GPUs install a recent cudatoolkit
package that works with the NVIDIA drivers on HPG (currently 12.x, but older versions are still supported) alongside the pytorch or tensorflow package(s). See RC provided tensorflow or pytorch installs for examples if needed. Mamba can detect if there is a gpu in the environment, so the easiest approach is to run the mamba install command in a gpu session. Alternatively, you can run mamba install on any node or if a cpu-only pytorch package was already installed by explicitly requiring a gpu version of pytorch when running mamba install. E.g.
mamba install cudatoolkit=11.3 pytorch=1.12.1=gpu_cuda* -c pytorch
Load the conda
module
Before we can run conda
or mamba
on HiPerGator, we need to load the conda
module:
module load conda
Create your environment
Create a name based environment
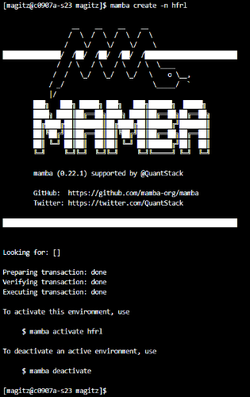
To create your first name based (see path based instructions below)conda
environment, run the following command. In this example, I am creating an environment named hfrl
:
mamba create -n hfrl
The screenshot to the right is the output from running that command. Yours should look similar.
Note:
- You do not need to manually create the folders that you setup in your
~/.condarc
file.mamba
will take care of that for you. - When creating a Conda environment you can also install Conda packages as needed at the same time. i.e:
mamba create -n hfrl python=3.9 pytorch numpy=2.22
Create a path based environment
To create a path based conda
environment use the '-p PATH' argument:
mamba create -p PATH
e.g.
mamba create -p /blue/mygroup/share/project42/conda/envs/hfrl/
Activate the new environment
To activate our environment (whether created with mamba
or conda we use the conda activate env_name
command. Let's activate our new environment:
conda activate hfrl
or
conda activate /blue/mygroup/share/project42/conda/envs/hfrl/
Notice that your command prompt changes when you activate an environment to indicate which environment is active, showing that in parentheses before the other information:
(hfrl) [magitz@c0907a-s23 magitz]$
Note: path based environment activation is really only needed for package installation. For using the environment just add the path to its bin
directory to $PATH in your job script.
Once you are done installing packages inside the environment you can use
$ conda deactivate
We do not recommend activating conda environments when _using_ them i.e. running programs installed in the environments. Please prepend the path to that environment to your $PATH instead.
E.g. If you have a project-specific conda environment at '/home/myuser/envs/project1/' add the following into your job script before executing any commands
export PATH=/home/myuser/envs/project1/bin:$PATH
Export or import an environment
Expand this section to view instructions.
Export your environment to an environment.yml
file
Now that you have your environment working, you may want to document its contents and/or share it with others. The environment.yml
file defines the environment and can be used to build a new environment with the same setup.
To export an environment file from an existing environment, run:
conda env export > hfrl.yml
You can inspect the contents of this file with cat hfrl.yml
. This file defines the packages and versions that make up the environment as it is at this point in time. Note that it also includes packages that were installed via pip
.
Create an environment from a yaml file
If you share the environment yaml file created above with another user, they can create a copy of your environment using the command:
conda env create --file hfrl.yml
They may need to edit the last line to change the location to match where they want their environment created.
Group environments
It is possible to create a shared environment accessed by a group on HiPerGator, storing the environment in, for example, /blue/group/share/conda
. In general, this works best if only one user has write access to the environment. All installs should be made by that one user and should be communicated with the other users in the group. It is recommended that user's umask configuration is set to group friendly permissions, such as umask 007. See Sharing Within A Cluster.
Install packages into your environment with mamba or pip
Expand this section to view instructions.
Now we are ready to start adding things to our environment.
There are a few ways to do this. We can install things one-by-one with either mamba install ____
or pip install ____
. We will look at using yaml files below.
pip install
will install the package into that environment. So, even if you continue using pip
, adding conda
environments solves the problem of everything being installed in one location--each environment has its own site-packages
folder and is isolated from other environments.mamba install
packages
Now we are ready to install packages using mamba install ___
.
Start with cudatoolkit
and pytorch/tensorflow
if using GPU!
To make sure your code will run on GPUs install a recent cudatoolkit
package that works with the NVIDIA drivers on HPG (currently 12.x, but older versions are still supported) alongside the pytorch or tensorflow package(s). See RC provided tensorflow or pytorch installs for examples if needed. Mamba can detect if there is a gpu in the environment, so the easiest approach is to run the mamba install command in a gpu session. Alternatively, you can run mamba install on any node or if a cpu-only pytorch package was already installed by explicitly requiring a gpu version of pytorch when running mamba install. E.g.
mamba install cudatoolkit=11.3 pytorch pytorch-cuda=11.3 -c pytorch -c nvidia
Load the conda
module
From the PyTorch Installation page, we should use:
mamba install pytorch torchvision torchaudio cudatoolkit=11.3 -c pytorch
When you run that command, mamba
will look in the repositories for the specified packages and their dependencies. Note we are specifying a particular version of cudatoolkit
. As of May, 2022, that is the correct version on HiPerGator.
mamba
will list the packages it will install and ask you to confirm the changes. Typing 'y' or hitting return will proceed; 'n' will cancel:
Finally, mamba
will summarize the results:
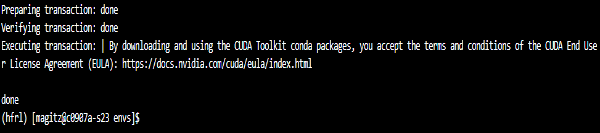
Tensorflow installation alternative
While not needed for this tutorial, many users will want TensorFlow instead of PyTorch, so we will provide the command for that here. To install TensorFlow, use this command:
mamba install tensorflow cudatoolkit=11.2
This post at conda-forge has additional information and tips for installing particular versions or installing on a non-GPU node: GPU enabled TensorFlow builds on conda-forge.
Install additional packages
This tutorial creates an environment for the Hugging Face Deep Reinforcement Learning Course, you can either follow along with that or adapt to your needs.
You can list more than one package at a time in the mamba install command. We need a couple more, so run:
mamba install gym-box2d stable-baselines3
Add packages to our environment with pip install
As noted above, not everything is available in a conda
channel. For example the next thing we want to install is huggingface_sb3
.
If we type mamba install huggingface_sb3
, we get a message saying nothing provides it as seen to the right:
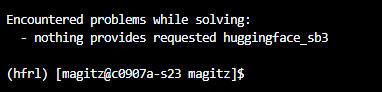
If we know of a conda
source that has that package, we can add it to the channels:
section of our ~/.condarc
file. That will prompt mamba
to include that location when searching.
But many things are only available via pip
. So...
pip install huggingface_sb3
That will install huggingface_sb3
. Again, because we are using environments and have the hfrl
environment active, pip
will not install huggingface_sb3
in our ~/.local/lib/python3.X/site-packages/ directory, but rather within in our hfrl
directory, at /blue/group/user/conda/envs/hfrl/lib/python3.10/site-packages
. This prevents the issues and headaches mentioned at the start.
Install additional packages
As with mamba
, we could list multiple packages in the pip install
command, but again, we only need one more:
pip install ale-py==0.7.4
Use your environment from command line or scripts
Now that we have our environment ready, we can use it from the command line or a script using something like:
module load conda conda activate hfrl # Run my amazing python script python amazing_script.py |
or with path based environments: |
# Set path to environment we want and pre-pend to PATH variable env_path=/blue/mygroup/share/project42/conda/bin export PATH=$env_path:$PATH # Run my amazing python script python amazing_script.py |
Setup a Jupyter Kernel for your environment
Expand this section to view instructions.
Often, we want to use the environment in a Jupyter notebook. To do that, we can create our own Jupyter Kernel.
Add the jupyterlab
package
In order to use an environment in Jupyter, we need to make sure we install the jupyterlab
package in the environment:
mamba install jupyterlab
Copy the template_kernel
folder to your path
On HiPerGator, Jupyter looks in two places for kernels when you launch a notebook:
/apps/jupyterhub/kernels/
for the globally available kernels that all users can use. (Also a good place to look for troubleshooting getting your own kernel going)~/.local/share/jupyter/kernels
for each user. (Again, your home directory and the.local
folder is hidden since it starts with a dot)
Make the ~/.local/share/jupyter/kernels
directory: mkdir -p ~/.local/share/jupyter/kernels
Copy the /apps/jupyterhub/template_kernel
folder into your ~/.local/share/jupyter/kernels
directory:
cp -r /apps/jupyterhub/template_kernel/ ~/.local/share/jupyter/kernels/hfrl
/apps/jupyterhub/kernels/
directory.Edit the template_kernel
files
The template_kernel
directory has four files: the run.sh
and kernel.json
files will need to be edited in a text editor. We will use nano
in this tutorial. The logo-64X64.png
and logo-32X32.png
are icons for your kernel to help visually distinguish it from others. You can upload icons of those dimensions to replace the files, but they need to be named with those names.
Edit the kernel.json
file
Let's start editing the kernel.json
file. As an example, we can use:
nano ~/.local/share/jupyter/kernels/hfrl/kernel.json
The template has most of the information and notes on what needs to be updated. Edit the file to look like:
{ "language": "python", "display_name": "HF_Deep_RL", "argv": [ "~/.local/share/jupyter/kernels/hfrl/run.sh", "-f", "{connection_file}" ] }
Edit the run.sh
file
The run.sh
file needs the path to the python
application that is in our environment. The easiest way to get that is to make sure the environment is activated and run the command: which python
The path it outputs should look something like: /blue/group/user/conda/envs/hfrl/bin/python
. Copy that path.
Edit the run.sh
file with nano
:
nano ~/.local/share/jupyter/kernels/hfrl/run.sh
The file should looks like this, but with your path:
#!/usr/bin/bash exec /blue/ufhpc/magitz/conda/envs/hfrl/bin/python -m ipykernel "$@"
If you are doing this in a Jupyter session, refresh your page. If not, launch Jupyter.
Your kernel should be available in the default kernel list ready for you to use!